OPEN-SOURCE SCRIPT
تم تحديثه [blackcat] L2 Ehlers Autocorrelation Periodogram V2
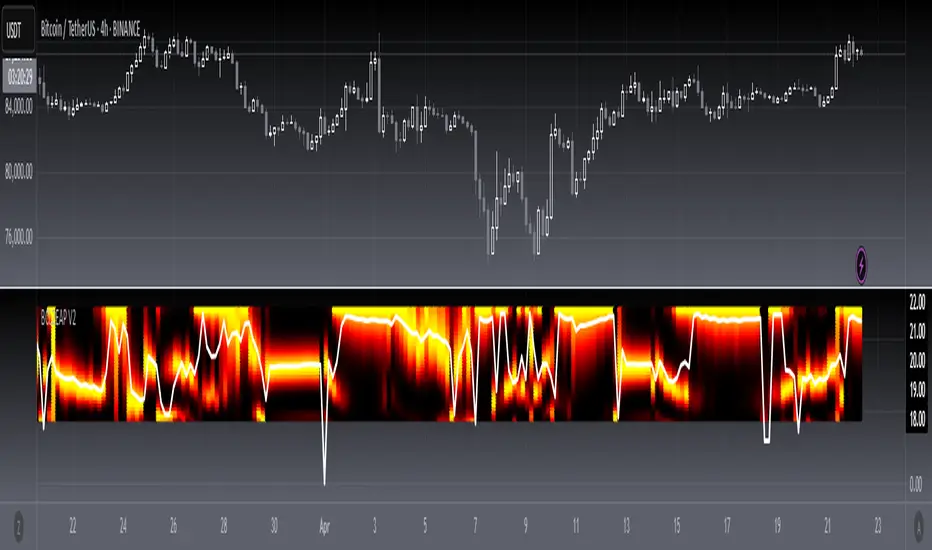
OVERVIEW
The Ehlers Autocorrelation Periodogram is a sophisticated technical analysis tool that identifies market cycles and their dominant frequencies using autocorrelation and spectral analysis techniques.
BACKGROUND
Developed by John F. Ehlers and detailed in his book "Cycle Analytics for Traders" (2013), this indicator combines autocorrelation functions with discrete Fourier transforms to extract cyclic information from price data.
FUNCTION
The indicator works through these key steps:
Calculates autocorrelation using minimum three-bar averaging
Applies discrete Fourier transform to extract cyclic information
Uses center-of-gravity algorithm to determine dominant cycle
ADVANTAGES
• Rapid response within half-cycle periods
• Accurate relative cyclic power estimation over time
• Correlation constraints between -1 and +1 eliminate amplitude compensation needs
• High resolution independent of windowing functions
HOW TO USE
Add the indicator to your chart
Adjust AvgLength input parameter:
• Default: 3 bars
• Higher values increase smoothing
• Lower values increase sensitivity
Interpret the results:
• Colored bars represent spectral power
• Red to yellow spectrum indicates cycle strength
• White line shows dominant cycle period
INTERPRETATION
• Strong colors indicate significant cyclic activity
• Sharp color transitions suggest potential cycle changes
• Dominant cycle line helps identify primary market rhythm
LIMITATIONS
• Requires sufficient historical data
• Performance may vary in non-cyclical markets
• Results depend on proper parameter settings
NOTES
• Uses highpass and super smoother filtering techniques
• Spectral estimates are normalized between 0 and 1
• Color intensity varies based on spectral power
THANKS
This implementation is based on Ehlers' original work and has been adapted for TradingView's Pine Script platform.
The Ehlers Autocorrelation Periodogram is a sophisticated technical analysis tool that identifies market cycles and their dominant frequencies using autocorrelation and spectral analysis techniques.
BACKGROUND
Developed by John F. Ehlers and detailed in his book "Cycle Analytics for Traders" (2013), this indicator combines autocorrelation functions with discrete Fourier transforms to extract cyclic information from price data.
FUNCTION
The indicator works through these key steps:
Calculates autocorrelation using minimum three-bar averaging
Applies discrete Fourier transform to extract cyclic information
Uses center-of-gravity algorithm to determine dominant cycle
ADVANTAGES
• Rapid response within half-cycle periods
• Accurate relative cyclic power estimation over time
• Correlation constraints between -1 and +1 eliminate amplitude compensation needs
• High resolution independent of windowing functions
HOW TO USE
Add the indicator to your chart
Adjust AvgLength input parameter:
• Default: 3 bars
• Higher values increase smoothing
• Lower values increase sensitivity
Interpret the results:
• Colored bars represent spectral power
• Red to yellow spectrum indicates cycle strength
• White line shows dominant cycle period
INTERPRETATION
• Strong colors indicate significant cyclic activity
• Sharp color transitions suggest potential cycle changes
• Dominant cycle line helps identify primary market rhythm
LIMITATIONS
• Requires sufficient historical data
• Performance may vary in non-cyclical markets
• Results depend on proper parameter settings
NOTES
• Uses highpass and super smoother filtering techniques
• Spectral estimates are normalized between 0 and 1
• Color intensity varies based on spectral power
THANKS
This implementation is based on Ehlers' original work and has been adapted for TradingView's Pine Script platform.
ملاحظات الأخبار
OVERVIEW📊 The [blackcat] L2 Ehlers Autocorrelation Periodogram V2 represents an advanced technical analysis tool operating at Level 2 precision. This sophisticated indicator builds upon Dr. John F. Ehlers' groundbreaking work from Chapter 8 of "Cycle Analytics for Traders", providing traders with deep insights into market cyclical patterns through refined spectral analysis techniques.
HISTORICAL CONTEXT
📚 Development Background
In 2013, Dr. John F. Ehlers revolutionized technical analysis by introducing the concept of autocorrelation periodograms in his seminal work "Cycle Analytics for Traders". His methodology merged traditional correlation analysis with modern spectral estimation techniques, offering a more accurate and responsive way to detect market cycles.
FUNDAMENTAL FUNCTIONALITY
🔄 Core Mechanisms
Autocorrelation Foundation
Begins with minimum three-bar averaging
Employs discrete Fourier Transform (DFT)
Captures spectral characteristics dynamically
Advanced Processing Features
Real-time spectral estimation
Dynamic cyclic power assessment
High-resolution cycle detection
SPECIFIC ADVANTAGES
💡 Unique Benefits
Speed & Responsiveness
Forms spectral estimates within half-cycle periods
Enables faster reaction to changing market conditions
Power Estimation Accuracy
Measures relative cyclic power over time
Differentiates between trending and cyclical phases
Provides nuanced power distribution across cycles
Amplitude Constraints
Maintains autocorrelation bounds (-1 to +1)
Eliminates need for spectral dilation compensation
Ensures consistent scaling across all cycle periods
Resolution Independence
Achieves high-resolution measurements
Performance unaffected by windowing functions
Delivers precise cycle identification
DETAILED OPERATIONAL PROCESS
⚙️ Technical Workflow
Preprocessing Stage
Implements high-pass filtering
Applies super smoother filter
Cleanses input data for accurate analysis
Correlation Calculation
Computes Pearson correlations (lags 0-48)
Stores intermediate results efficiently
Filters out weak/irrelevant correlations
Spectral Transformation
Transforms correlations via DFT
Extracts frequency components
Normalizes power spectrum
Dominant Cycle Detection
Utilizes Center-of-Gravity (CG) algorithm
Calculates weighted average periods
Identifies primary market cycles
VISUAL REPRESENTATION
🎨 Color Coding System
High Power Regions (>0.5)
Combines red and yellow hues
Yellow indicates maximum power (1.0)
Red marks moderate power (0.5)
Low Power Regions (<0.5)
Decreases red saturation
Approaches black at minimal power (0.0)
Indicates weaker cyclical influence
PRACTICAL APPLICATION GUIDELINES
🎯 Usage Framework
Initial Setup
Configure AvgLength parameter optimally
Enable appropriate overlay settings
Integrate with complementary indicators
Interpretation Process
Analyze color transitions systematically
Monitor dominant cycle variations
Look for consensus among multiple readings
Trade Implementation
Time entries during established cycles
Anticipate reversals using power changes
Manage positions based on cycle strength
RISK MANAGEMENT CONSIDERATIONS
🛡️ Safety Protocols
Signal Validation
Require multi-period confirmation
Cross-reference with additional indicators
Consider broader market context
Position Management
Adjust sizes according to cycle strength
Implement proper stop-loss placement
Maintain disciplined risk parameters
Emergency Procedures
Have predefined exit strategies ready
Set clear loss limits
Practice quick adaptation when needed
TROUBLESHOOTING AND OPTIMIZATION
🛠️ Fine-Tuning Guide
Parameter Optimization
Test different AvgLength settings
Validate configurations historically
Document successful implementations
Market-Specific Customization
Adjust for varying volatility levels
Account for different timeframe behaviors
Fine-tune based on asset class properties
Performance Tracking
Monitor signal reliability
Compare results across instruments
Regularly update strategy parameters
THEORETICAL FOUNDATIONS
🔍 Mathematical Principles
Center-of-Gravity Algorithm
Computes weighted average periods
Determines dominant cycle length
Updates continuously with new data
Normalized Spectrum Values
Ranges from 0 to 1
Maps directly to visual representation
Facilitates intuitive interpretation
AUTHORS AND ACKNOWLEDGMENTS
🌟 Special thanks to:
Dr. John F. Ehlers for pioneering research
نص برمجي مفتوح المصدر
بروح TradingView الحقيقية، قام مبتكر هذا النص البرمجي بجعله مفتوح المصدر، بحيث يمكن للمتداولين مراجعة وظائفه والتحقق منها. شكرا للمؤلف! بينما يمكنك استخدامه مجانًا، تذكر أن إعادة نشر الكود يخضع لقواعد الموقع الخاصة بنا.
للوصول السريع إلى الرسم البياني، أضف هذا النص البرمجي إلى مفضلاتك - تعرف على المزيد هنا.
Avoid losing contact!Don't miss out! The first and most important thing to do is to join my Discord chat now! Click here to start your adventure: discord.com/invite/ZTGpQJq 防止失联,请立即行动,加入本猫聊天群: discord.com/invite/ZTGpQJq
إخلاء المسؤولية
لا يُقصد بالمعلومات والمنشورات أن تكون، أو تشكل، أي نصيحة مالية أو استثمارية أو تجارية أو أنواع أخرى من النصائح أو التوصيات المقدمة أو المعتمدة من TradingView. اقرأ المزيد في شروط الاستخدام.
نص برمجي مفتوح المصدر
بروح TradingView الحقيقية، قام مبتكر هذا النص البرمجي بجعله مفتوح المصدر، بحيث يمكن للمتداولين مراجعة وظائفه والتحقق منها. شكرا للمؤلف! بينما يمكنك استخدامه مجانًا، تذكر أن إعادة نشر الكود يخضع لقواعد الموقع الخاصة بنا.
للوصول السريع إلى الرسم البياني، أضف هذا النص البرمجي إلى مفضلاتك - تعرف على المزيد هنا.
Avoid losing contact!Don't miss out! The first and most important thing to do is to join my Discord chat now! Click here to start your adventure: discord.com/invite/ZTGpQJq 防止失联,请立即行动,加入本猫聊天群: discord.com/invite/ZTGpQJq
إخلاء المسؤولية
لا يُقصد بالمعلومات والمنشورات أن تكون، أو تشكل، أي نصيحة مالية أو استثمارية أو تجارية أو أنواع أخرى من النصائح أو التوصيات المقدمة أو المعتمدة من TradingView. اقرأ المزيد في شروط الاستخدام.